Algorithmic Trading Hedge Funds: Past, Present, and Future
Hedge funds have seen dramatic growth since starting at a mere $100,000 in total assets more than 70 years ago.1 The number of hedge funds globally has increased to around 8,000,2 now holding a total asset value of more than $4 trillion – an all-time high.3 And after a difficult period from 2008 to 2020, during which 11,600 hedge funds closed down, they’re incredibly popular with investors once more. In 2021, a record 1,144 hedge funds stopped accepting new investments because they had enough money.4
Within this competitive environment, the investment strategies of top-performing hedge funds are increasingly dominated by algorithmic trading, with more than 50 percent of hedge funds now employing algorithms to trade the majority of their total value traded.5 To come to grips with this development, it’s important to take a step back and understand how the industry got to this point.
A new approach to investing
Born to a U.S. diplomat in Australia in 1901, Alfred Winslow Jones read for his Doctorate in Sociology at Columbia University in 1941. He went on to work as a reporter for Fortune magazine. Despite not having much experience covering financial topics, he was assigned to write an article on “Fashions in Forecasting”. It was during his research for this assignment that Jones realized he’d uncovered a novel and productive approach to investing. By combining the advantages of long and short selling, Jones created a type of fund he believed could serve risk-averse investors and the public good.6
His strategy was to balance long positions (purchasing a share at a future time at a pre-agreed, higher price and set volume) while selling short other stocks (agreeing to a lower price and on a set volume in the future). The hedge fund could offset risk on their portfolio by betting on both longs and shorts on the same or related stocks.7 Debt raised against the fund assets (leverage) combined with this risk offset mechanism gave him a new perspective on how to use pooled investment resources and paved the way to a new trading philosophy.
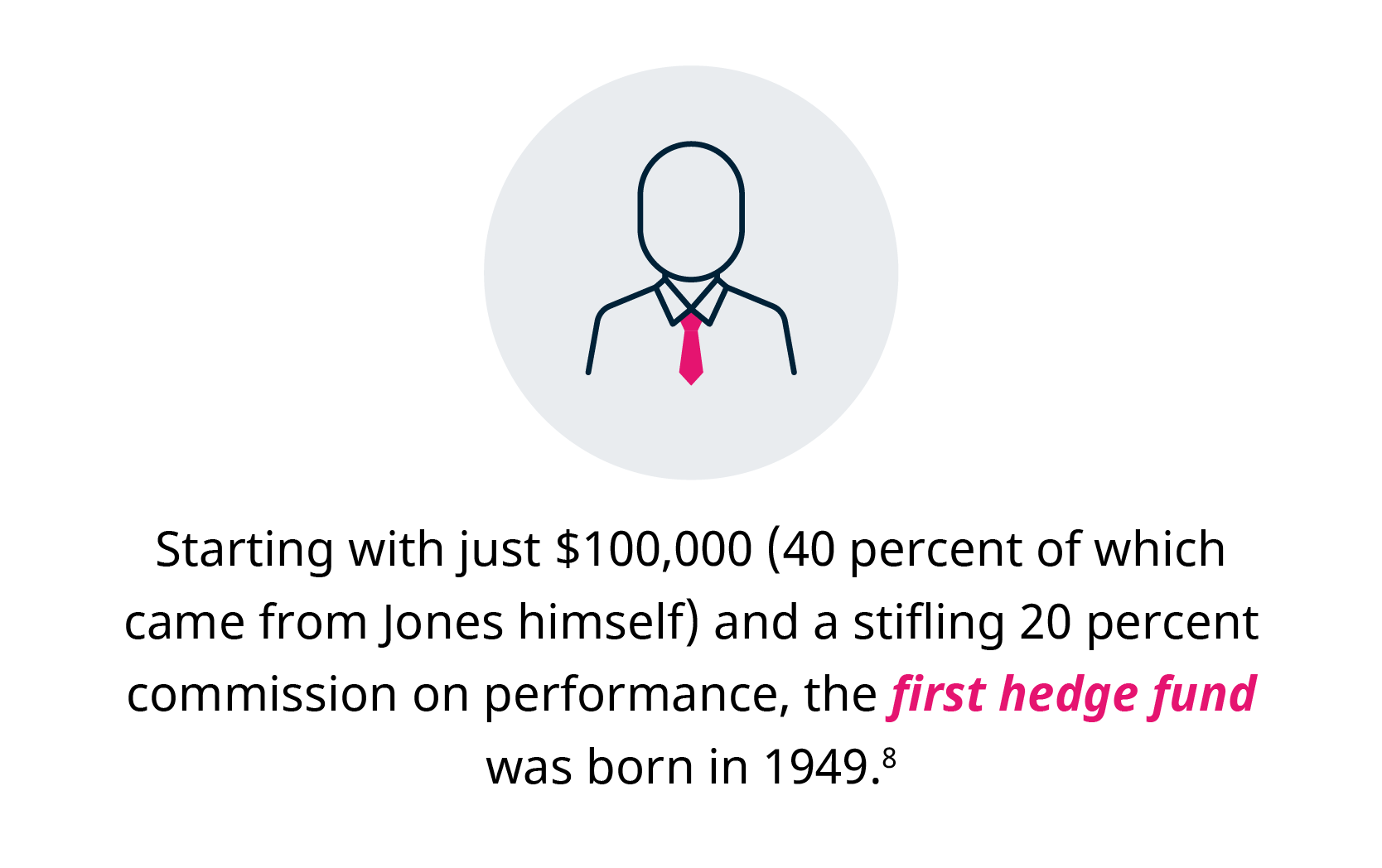
Jones and his team quietly outperformed the market for years, achieving 5,000 percent return by 1968.9 It wasn’t until 1966, when another Fortune article noted Jones’s fund had outperformed the top Dreyfus Fund by a staggering 87 percent over 10 years, that serious competitors started joining the market.10
A hedge fund, therefore, became a partnership between various investors where the fund pools assets, attempts to leverage it to borrow further funds, and uses various strategies (such as derivatives, futures, etc.) to earn active returns off both local and international markets.11
What is algorithmic trading?
Algorithmic trading is the use of algorithms or rules to make purchasing and sales decisions on the investor’s behalf, based on a set of instructions defining price, quantity, timing, or any mathematical model.12 This type of trading maximizes returns by arbitraging the advantages of enormous amounts of data, tiny processing delays, huge throughput capacity, dynamically balanced portfolios, and intelligent predictive analytics. By exploiting one or more of these systemic improvements, algorithmic trading can take many forms: trading ahead of index fund rebalancing, arbitrage, scalping and mean reversion, pairs trading, and delta-neutral strategies. Irrespective of the strategy, algorithmic trading almost always means reduced transaction costs for the investor.13
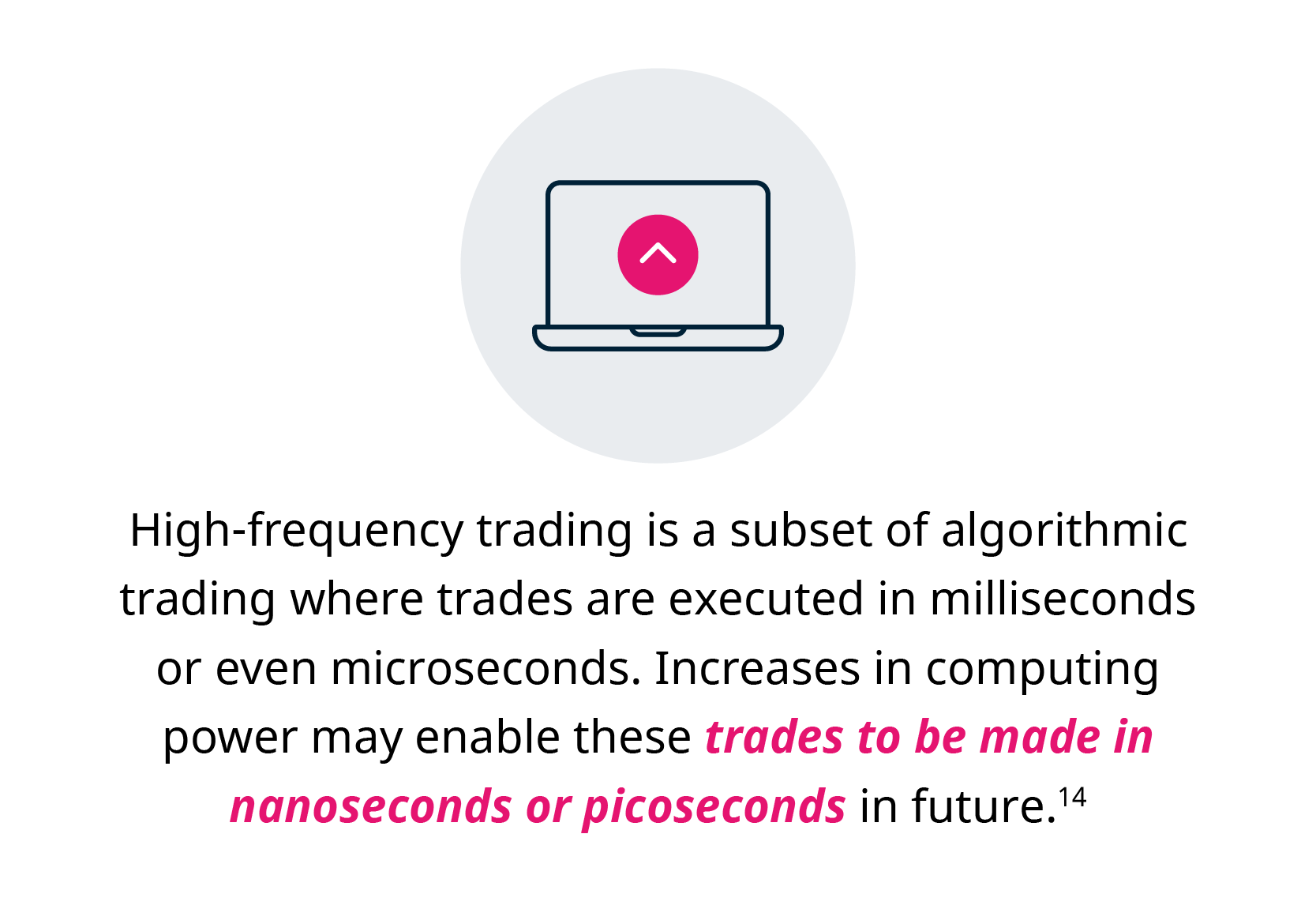
Computerized trading systems were introduced in U.S. financial markets in the 1970s. These systems included the Designated Order Turnaround system, which increased efficiency by routing orders for listed securities directly to a trading floor specialist, bypassing the broker.15 The increasing digitization of stock markets throughout the 1980s and 1990s changed the structure of stock trading. Algorithmic trading proliferated in the market due to the U.S. Securities and Exchange Commission authorizing electronic exchanges in 1998.16 Decimalization of the stock market in 2005 decreased the minimum tick size (the minimum price increment change of a trading instrument17) from 6.5 cents to 1 cent. This increased the volume of algorithmic trades because of the reduced costs involved in buying and selling smaller-increment stocks at high speed.18
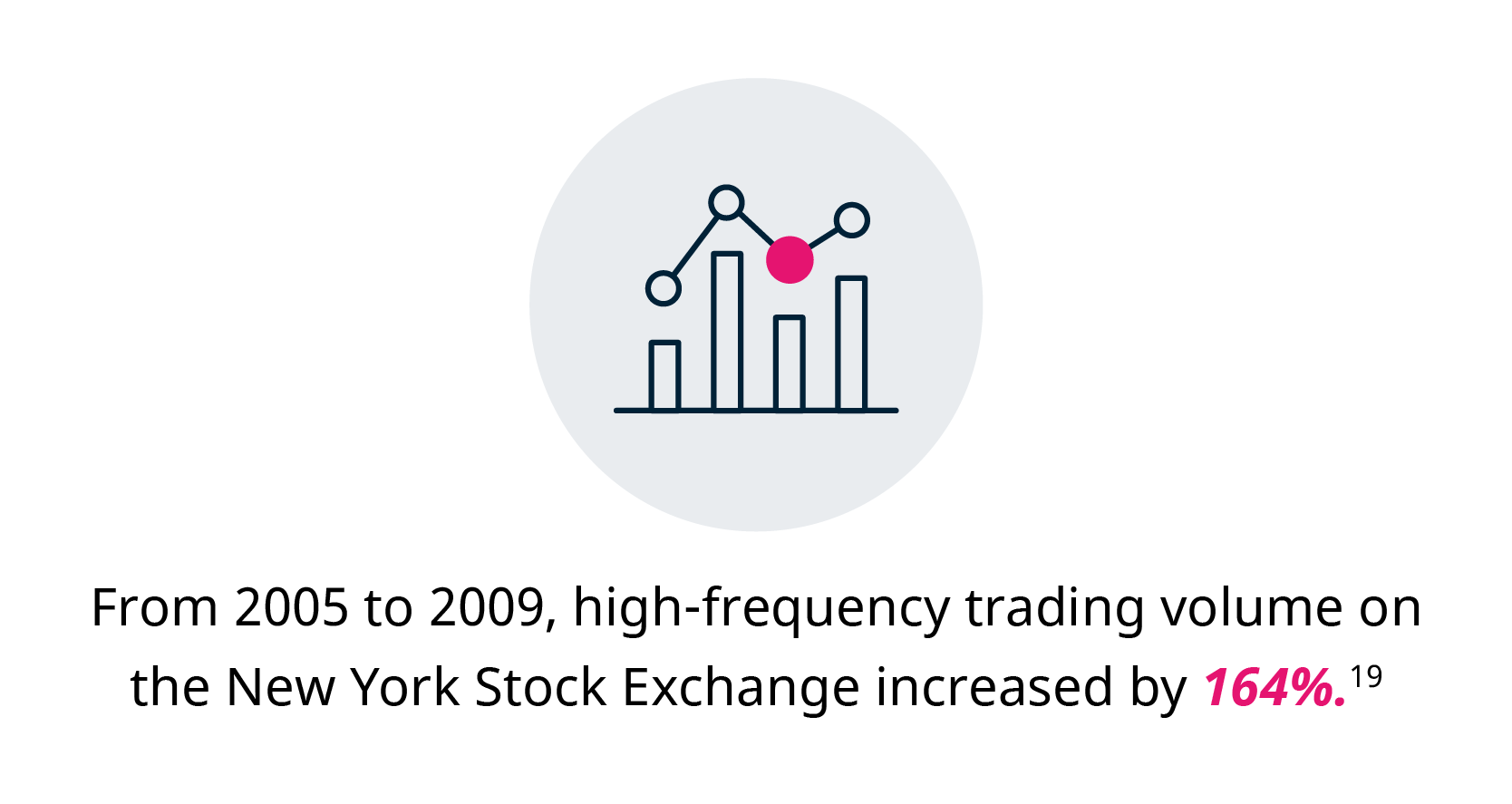
However, algorithmic trading isn’t without its unique risks and inherent problems. The method has been blamed for a number of stock exchange crashes and some monumental losses.
On May 6, 2010, the Dow Jones Industrial went into chaos in what has since been dubbed the ‘Flash Crash’, losing nearly 1,000 points in minutes – the most significant loss in its history. In total, around $1 trillion was wiped off market value.20
After much investigation, individual trader Navinder Singh Sarao was eventually convicted for his role in the crash. He was found to have used algorithms to place thousands of orders to sell e-mini S&P contracts, then canceling the trades and buying them at lower prices.21 This anomaly was accentuated by other algorithmic traders trying to adjust and respond.22
Two years later, Knight Capital provided the world with another discouraging mishap. Immediately after taking their new algorithmic trading platform live in 2012, they realized something was wrong with their high-frequency trading strategies. The software bought and sold $7 billion of shares on the New York Stock Exchange at bad prices, costing the firm $440 million – 40 percent of its value.23
Algorithmic trading and hedge funds today
Fortunately, since then, algorithmic trading has evolved into a market-leading solution. Accompanying infrastructure has been developed to facilitate high-frequency trading, including high-speed computers co-located as close as possible to the exchange servers and real-time data feeds. This form of trading has reduced bid-ask spreads (the difference between the asking price and the offering price of a security or other asset), making markets more efficient, increasing liquidity, and assisting in the price discovery and formation process.24
Considering the possibilities offered by advances in technology and software, contemporary funds are increasingly turning to quantitative or systematic analysis based on algorithms and computer models. Algorithmic hedge funds are commonly known as quant funds.25
Quant fund managers input data into computer programs that analyze it and systematically carry out investment strategies based on pre-defined trading signals. Trading positions are based on factors such as economic data points, new reports, or price trends in asset values.26
One of the selling points of algorithmic trading is that it’s supposedly not subject to cognitive bias – systematic deviations from rational behavior that typically result in worse investment performance. However, a study published by Harvard Business Review found that while algorithms achieved a better internal return rate (7.26 percent) than novice angel investors (2.56 percent), highly experienced investors still achieved significantly higher returns (22.75 percent).27
Artificial intelligence (AI) and machine learning (ML) algorithms are also being deployed on the market, with limited success. A University of Cambridge study found that the few AI-driven funds that publicly posted their performance data underperformed in comparison with their human counterparts, making a case for the continued involvement of human analysts and managers. The authors pointed to a hybrid model as the best approach, embedding AI in support and analytics tools while leaving decision-making up to humans.28
The future of algorithmic trading
With robo-advisors and hedge funds wielding algorithms like magic wands, there’s increased pressure on financial professionals to stay relevant.
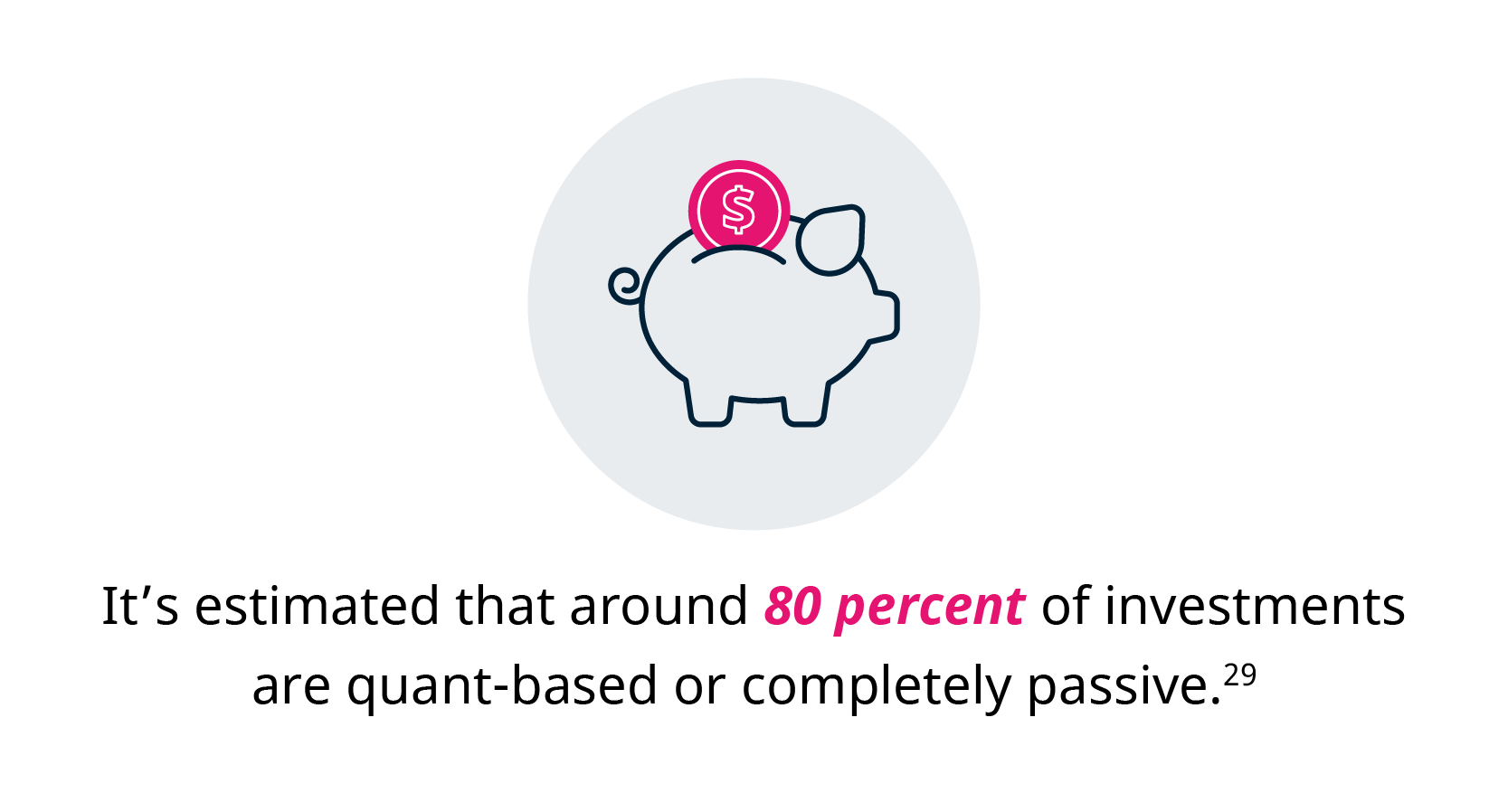
Competing with the sheer processing capacity and 20-nanosecond latency of huge data centers is humanly impossible. Innovative application of these technologies and creative problem-solving will be required from corporates and start-ups alike should they wish to ensure market relevance in the coming years. In particular, proactive and forward-looking businesses will be paying attention to the types of data they can apply by means of collecting, processing (with the help of ML trading), and leveraging it for intelligent (human-assisted) investment decisions.
Following initial efforts by the stock market to make their systems open via (an early form) application programming interface (API) in 1998, there have been examples of this thinking permeating other components of the financial sector. Within the EU, for example, Payment Services Directive 2 (PSD2) is a piece of regulation that compels financial institutions to provide secure, privileged, and consenting access to banking details by third parties via an API.30 Its fundamental purpose is to reduce systemic friction and empower a new wave of products and services built upon existing banking infrastructure. This sort of thinking is expected to eventually extend into all areas of the financial market – further exposing opportunities for agile, proactive financial market players.
Algorithms and the tools driving algorithmic trading are increasingly available to the amateur investor, further accentuating pressure on industry leaders to stay relevant. And while individual investors may not have the resources necessary to run sophisticated portfolio-management systems, there’s a plethora of accessible online courses and tools available for those interested in creating algorithms and implementing their own trading strategies. There are also growing and enthusiastic communities on platforms like Reddit that share information and links to tools and resources.31
Demystify algorithmic trading and develop the ability to implement your own trading strategies with the Oxford Algorithmic Trading Programme from Saïd Business School, University of Oxford.
The success trifecta that characterizes algorithmic trading – strong performance, broad adoption, and relatively low workforce needs – also presents an interesting situation for individual financial market employees. GetSmarter’s The Future of Work is Here report found that among respondents in financial services, technology and automation are seen by 25 percent and 15 percent respectively as key drivers of change.
As we’ve seen, however, the human element remains critical in making complex financial judgments, particularly in the context of disruptions like the COVID-19 pandemic. People also remain essential to relationship building, system development, and various other critical functions within the business. Leveraging algorithmic tools to focus on execution provides key advantages in certain capacities, and enables employees to focus on building truly innovative, disruptive solutions to future problems.
Adapting to a future of change
The 21st century is categorized by an increasing rate of change, growing levels of uncertainty, and greater reliance on and integration of technology into societies, cultures, and workplaces.32
To become or remain relevant, today’s businesses must clearly identify their purpose, understand their new role, keep up to date with developments, and stay agile to environmental and market forces. Driven by innovation and disruption, this shifting environment will present many groundbreaking opportunities for rewarding careers in trading. Preparation and adaptability will ultimately determine who thrives.
Gain technical and financial insight with an online short course
- 1 Gad, S. (Apr, 2021). ‘Guide to hedge funds’. Retrieved from Investopedia.
- 2 Kumar, N. (Aug, 2021). ‘Hedge funds are hot again. Good luck finding one that’ll take your money’. Retrieved from Bloomberg.
- 3 (Jan, 2022). ‘Global hedge fund industry assets top $4 trillion for the first time’. Retrieved from Reuters.
- 4 Kumar, N. (Aug, 2021). ‘Hedge funds are hot again. Good luck finding one that’ll take your money’. Retrieved from Bloomberg.
- 5 (Jul, 2021). ‘Hedge funds rely on algos to trade majority of portfolios in market stress’. Retrieved from The Trade.
- 6 Huyssen, D. (Feb, 2021). ‘Op-Ed: What would the socialist who created the hedge fund think of the GameStop mess?’. Retrieved from LA Times.
- 7 DeMatos, D. (Mar, 2021). ‘TCH: Alfred Winslow Jones and the first hedge fund’. Retrieved from LinkedIn.
- 8 DeMatos, D. (Mar, 2021). ‘TCH: Alfred Winslow Jones and the first hedge fund’. Retrieved from LinkedIn.
- 9 DeMatos, D. (Mar, 2021). ‘TCH: Alfred Winslow Jones and the first hedge fund’. Retrieved from LinkedIn.
- 10 Zainul-Abidin, S. (Nov, 2020). ‘The hedge fund evolution’. Retrieved from Singapore Management University.
- 11 Gad, S. (Apr, 2021). ‘Guide to hedge funds’. Retrieved from Investopedia.
- 12 Seth, S. (May, 2021). ‘Basics of algorithmic trading: Concepts and examples’. Retrieved from Investopedia.
- 13 Seth, S. (May, 2021). ‘Basics of algorithmic trading: Concepts and examples’. Retrieved from Investopedia.
- 14 Seth, S. (Dec, 2021). ‘The world of high-frequency algorithmic trading’. Retrieved from Investopedia.
- 15 Kenton, W. (Oct, 2021). ‘Designated Order Turnaround (DOT (SuperDOT))’. Retrieved from Investopedia.
- 16 Seth, S. (Dec, 2021). ‘The world of high-frequency algorithmic trading’. Retrieved from Investopedia.
- 17 Segal, T. (May, 2021). ‘Tick size definition’. Retrieved from Investopedia.
- 18 Andrews, E. (Aug, 2021). ‘The fast and the incurious: How high-speed stock trading pushes out smart money’. Retrieved from Stanford Graduate School of Business.
- 19 Bennur, S. (Jan, 2021). ‘Evolution of algorithmic trading and assessing the impact of it on capital market’. Retrieved from ResearchGate.
- 20 (Aug, 2021). ‘The flash crash of 2010’. Retrieved from AVA Trade.
- 21 (Aug, 2021). ‘The flash crash of 2010’. Retrieved from AVA Trade.
- 22 (Aug, 2020). ‘Staff Report on Algorithmic Trading in U.S. Capital Markets’. Retrieved from U.S. Securities and Exchange Commission.
- 23 Seth, S. (Dec, 2021). ‘The world of high-frequency algorithmic trading’. Retrieved from Investopedia.
- 24 Seth, S. (Dec, 2021). ‘The world of high-frequency algorithmic trading’. Retrieved from Investopedia.
- 25 Price, M. (Jan, 2022). ‘What is a quant fund?’. Retrieved from The Balance.
- 26 (Nov, 2021). ‘Demystifying quant hedge funds’. Retrieved from Crystal Capital Partners.
- 27 Antretter, T., et al. (Nov, 2020). ‘Do algorithms make better – and fairer – investments than angel investors?’. Retrieved from Harvard Business Review.
- 28 Sahakian, B., et al. (Feb, 2022). ‘Humans v AI: here’s who’s better at making money in financial markets’. Retrieved from The Conversation.
- 29 Puutio, A. (Jun, 2021). ‘How a group of Redditors is making algotrading accessible to all’. Retrieved from Forbes.
- 30 Russo, D. (Jul, 2021). ‘Payments and market infrastructure two decades after the start of the European Central Bank’. Retrieved from European Central Bank.
- 31 Puutio, A. (Jun, 2021). ‘How a group of Redditors is making algotrading accessible to all’. Retrieved from Forbes.
- 32 De Smet, A., et al. (Jan, 2021). ‘Organizing for the future: Nine keys to becoming a future-ready company’. Retrieved from McKinsey.