Data Analysis and the Importance of Analytical Tools
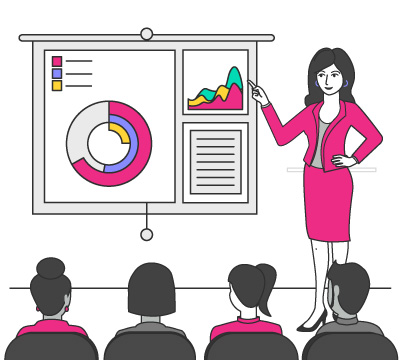
We are awash in data: 90% of all data was created in the last two years, an amount of facts and statistics so vast as to be quite literally unfathomable.1 Yet, for business, there exists enormous opportunity in extracting meaning from the incredible volumes of data created every minute.
This job falls to data analysts and as a consequence, between 2016 and 2026, demand for data analysts and other similar occupations is projected to grow 33%.2
That begs the question: why are data analysts seen to hold the key to unlocking the potential profit buried in data, and what tools do they need?
What does a data analyst do?
In the simplest terms, a data analyst is an interpreter.
In the simplest terms, a data analyst is an interpreter. They take raw data and turn it into meaningful insights that businesses can use for more effective decision-making. A data analyst can cover a number of fields, including finance, marketing and operations, and their findings could, for instance, result in:
- Reduced transportation costs
- Insights into customer behaviour
- Early warnings of adverse market conditions4
The insights data analysts provide can have an outsized impact on an organisation, potentially giving it a competitive edge5 that could increase its profitability. However, it’s not enough for data analysts to interpret data – they also need certain soft skills to be successful in the field. Data analysts should be able to communicate the insights they gain, otherwise it will be difficult for a business to act effectively on their findings. They also have to be creative thinkers to be able to derive useful insights from raw data.6
It’s almost impossible to overstate the importance of a data analyst in a business. According to Forbes, “85% of companies that are succeeding with analytics (i.e. those organisations that consider themselves to be analytics pacesetters in their markets and have an enterprise-wide analytics strategy in place) are seeing revenue growth greater than 7%”.7
However, no data analyst can work in isolation. To be effective, they must have the right tools that will allow them to parse through reams of data and derive useful intelligence from it.
After all, a carpenter needs tools to turn a pile of lumber into a couch, chair or table. Without those tools, the lumber will remain nothing but glorified firewood. Data analysts might not need a hammer, but without the right tools, they’ll be unable to extract anything meaningful from the enormous volumes of data available to businesses.
Here are five tools data analysts use to extract the insights a company needs:
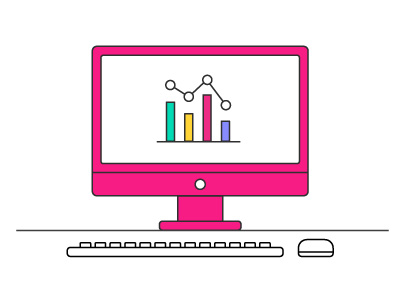
Excel
Excel – and its Google-developed cousin, Sheets – is not specifically meant for statistical analysis, but still plays an important supporting role in data analytics and is often the first stop for those entering the field. While it can perform certain analyses, particularly in the initial stages, Excel struggles with larger data sets.8
Tableau
Tableau offers a high level of flexibility, while remaining fairly simple to use.
Able to perform end-to-end data analytics through its platform as well as a suite of other offerings,9 Tableau offers a high level of flexibility, while remaining fairly simple to use.10 Of particular value is its ability to handle large amounts of data, generate interactive reports and provide embedded analytics.
SAS
The oldest entry on our list, SAS – the Statistical Analysis System – began development in 1966 and its longevity is earned. This software programme’s share of the global predictive analytics market stood at more than 30% in 2017.11 Although not ideal for visualisation, SAS provides a large number of statistical and data-analysis functions as well as excellent supporting tools.12
Python
Created in 1991, Python is a high-level coding language which is very popular with data scientists, thanks to its ease of use and versatility.13 On the downside, it does have a reputation for being a little slow,14 and despite its relative simplicity, users require some programming knowledge to use it to any effect.
R
Unlike Python, R is a programming language that was developed specifically to perform statistical analyses. Along with Python, it’s the most popular language used in data science and analytics.15 Where Python shines in its ability to manipulate data and perform repeated tasks, R is more appropriate for once-off data deep dives and statistical heavy lifting.16
R, like Python, also requires extensive coding knowledge, and is perhaps a little less versatile, given its narrow focus. Neither language is suitable for beginners, but hold great benefits for experienced data analysts who want to develop their skills and are willing to put in the time to learn the language first.
If you’re interested in becoming a data analyst, or are a manager looking for ways to leverage big data in your organisation, consider taking part in an online short course, and start developing your knowledge.
- 1 Marr, B. (May, 2018). ‘How Much Data Do We Create Every Day? The Mind-Blowing Stats Everyone Should Read’. Retrieved from Forbes.
- 2 (Apr, 2018). ‘Occupational Outlook Handbook: Mathematicians and Statisticians’. Retrieved from U.S. Bureau of Labor Statistics.
- 3 (Nd). ‘Data Analyst Job Description’. Retrieved from Snag.
- 4 (Nd). ‘Data Analyst Job Description’. Retrieved from Snag.
- 5 (Aug, 2018). ‘6 Reasons Why Investment In Analytics Is Essential’. Retrieved from Forbes.
- 6 Gutierrez, D. (Aug, 2017). ‘5 Skills You Need to Become a Data Analyst’. Retrieved from Inside Big Data.
- 7 (Aug, 2018). ‘6 Reasons Why Investment In Analytics Is Essential’. Retrieved from Forbes.
- 8 Ray, S. (Nov, 2015). ‘Simple Yet Powerful Excel Tricks for Analyzing Data’. Retrieved from Analytics Vidhya.
- 9 (Nd). ‘What is Tableau?’ Retrieved from Tableau.
- 10 Bell, S. (Apr, 2018). ‘Top 15 Data Analytics Tools’. Retrieved from import.io.
- 11 Vesset, D, Gopal, C, Olofson, CW, Bond, S, Fleming, M, Schubmehl, D. (2018). ‘Worldwide Big Data and Analytics Software 2017 Market Shares’ [PDF]. Retrieved from SAS.
- 12 ‘SAS vs R – Which One Is Better for You?’ Retrieved from BusinessTown.
- 13 Gall, R. (Oct, 2017). ‘Should you use Python for data science?’. Retrieved from ITProPortal.
- 14 Yegulalp, S. (Jun, 2018). ‘What is Python? Everything you need to know’. Retrieved from InfoWorld.
- 15 Kopf, D. (Sep, 2017). ‘If you want to upgrade your data analysis skills, which programming language should you learn?’ Retrieved from Quartz.
- 16 Kopf, D. (Sep, 2017). ‘If you want to upgrade your data analysis skills, which programming language should you learn?’ Retrieved from Quartz.