The Future of Data Science
The digital age we live in is changing the way that we work, play, and learn. For organisations striving to achieve digital transformation, or behave more competitively through data analytics, becoming data-driven is a key goal. The progress in achieving this goal is dishearteningly slow.
According to the 2019 New Vantage Partners Big Data and AI Executive Survey, almost 72 per cent of the executives from organisations surveyed, such as American Express, Ford Motor, General Electric, General Motors, and Johnson & Johnson, believe that they have not successfully created a data culture.1 Only 31 per cent say that their businesses are data-driven, while 53 per cent report that they have not started to view data as a business asset. More than half (52 per cent) of the respondents confess that they are not successfully competing on data and analytics.
With the increasing amount of data available to organisations, businesses are becoming more urgent about the need to invest in data scientists.2
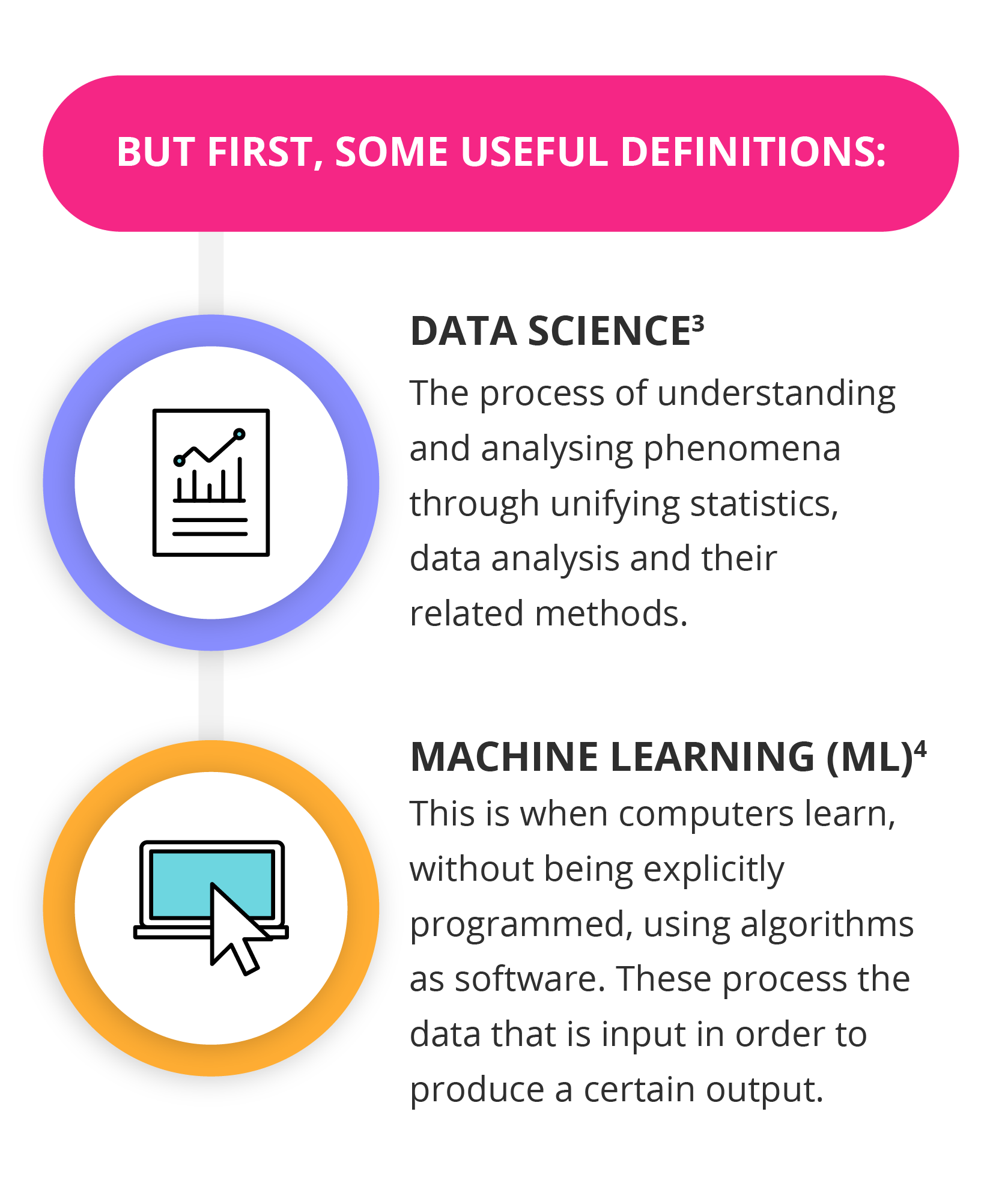
The explosion of data
Since the onset of the digital era, data is continuing to grow at an exponential rate, with very little sign of slowing down.5 This constant influx of data acts as fuel for existing data science models, creating a breeding ground for improved data science models, as well as creative, fresh use cases.
The growth of Internet of Things (IoT).6 A large reason for this data explosion is IoT. Around seven billion devices are connected IoT around the world, and it’s predicted to grow to a staggering 21.5 billion in seven years. It is expected that the airline, mining, and auto industries will be the biggest contributors in IoT data, and, as the quality of device technology continues to improve, the type of data harvested will become richer and more diversified.
Social Media. Facebook saw an average of 1.56 billion daily active users in March 2019 alone, with a total of 2.38 billion monthly active users by the end of that month.7 YouTube reports over a billion hours of video watched daily on their platform, with over 1.9 billion logged-in users visiting every month.8 Every time a person signs in to view their newsfeed or watch videos, data is generated – age, gender, location, language, duration of views, types of videos watched, type of content liked and shared, user preferences, and more. These are the top two social media platforms used in the world, however there are many more:9
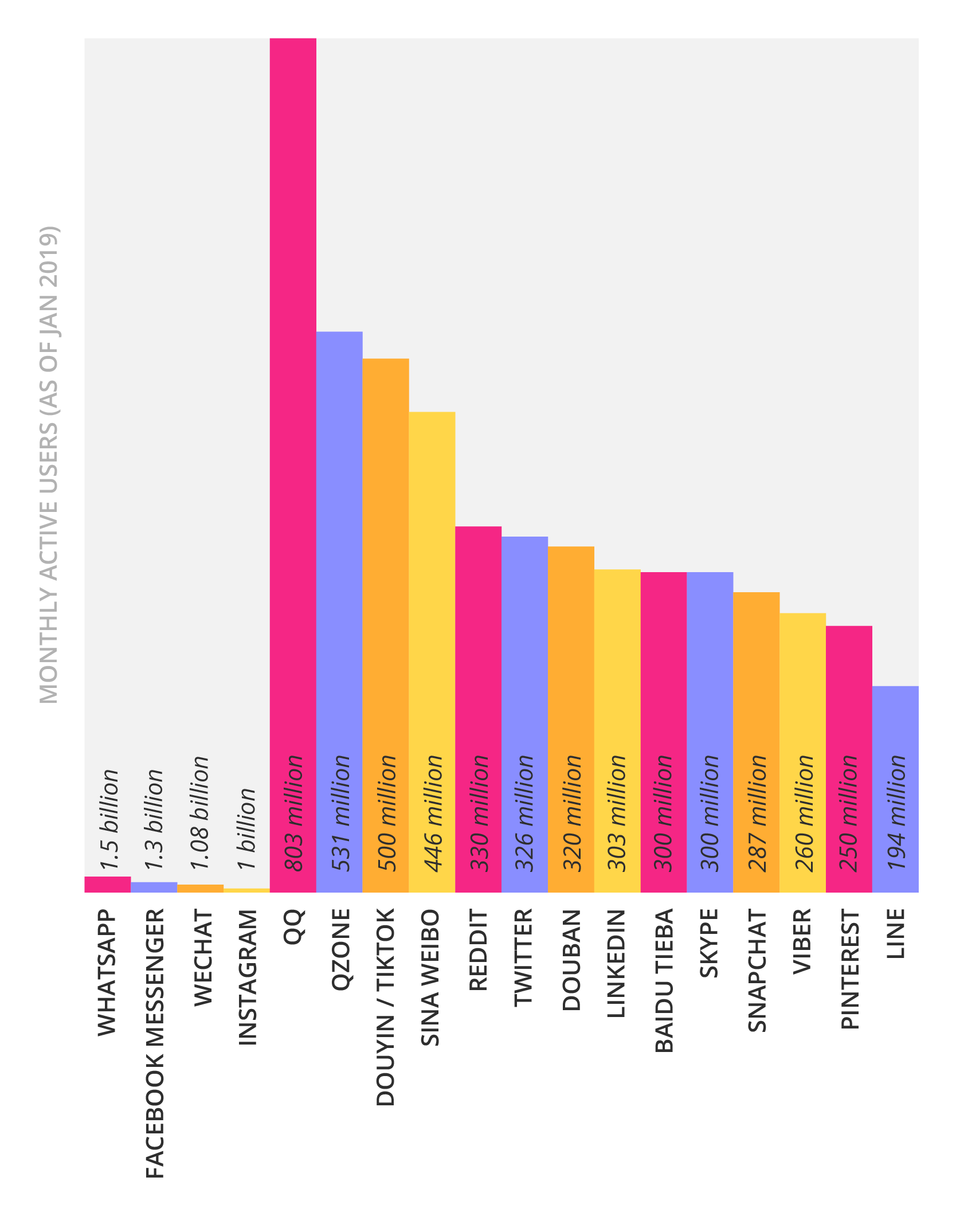
The sources of data will continue to expand as the need to harvest even greater amounts of information on customers grows. The greater access organisations have to data, the more precise and accurate the machine learning models will become. The potential applications of this data will further drive the need for data science.9 Without data science, it is difficult for organisations to access the full value from data.10
The rise of the machines
ML algorithms have seen a quick rise over the last few years, and with it, a focused development of ML software.12 This software is lowering the barriers to entry for those who wish to become data scientists.
The demand for data scientists is greater than can be supplied, with Bloomberg declaring it America’s hottest job in 2018.13 However, automation and education are making data science, research, and analytics more available to businesses.14 With a view to widen and accelerate the adoption of data science and analytics in business, most players in the data science field have made tool simplification a top priority. Coupled with a host of training resources that enable professionals from all backgrounds to gain much-needed data science skills, these skills are now more available to all industries.
These five factors are contributing to putting these all-important capabilities in the hands of more professionals, and potentially relieving a worldwide shortage of talent.
- Automated ML.15 Some estimate that data scientists spend close to 80 per cent of their day on repetitive tasks that could be fully or partially automated. Automating tasks such as data preparation, feature engineering and selection, and algorithm selection and evaluation, will help data scientists be more productive and effective.
- Low- or no-code app development.16 Various low-code and no-code software development platforms are available for IT and non-technical staff to develop AI apps, and could speed up the development and delivery of apps by up to 10 times faster than more traditional methods, thanks to their graphical user interfaces, drag-and-drop modules, and other user-friendly structures.
- Pre-trained AI models. Developing and training ML modules are part of the key deliverables of data scientists. Now, pre-trained AI models are effectively productising ML expertise and reducing the time and effort needed for training,17 some of which can even produce immediate key insights.18
- Self-service data analytics. Many business intelligence and analytics suppliers are making it possible for business or nontechnical users to access data-based insights without the use of data scientists. Salesforce,19 Adobe Analytics,20 Microsoft Power BI,21 and others offer self-service analytics tools to supplement data analytics and discovery. The ability to carry out natural language query and search, visual data discovery, and natural language generation gives business users quick access to insights, and helps them communicate data findings such as correlations, exceptions, clusters, links, and predictions.
- Accelerated learning.22 The availability of short-term training courses on data science, data science research, ML and AI for professionals with basic mathematics and coding backgrounds is accelerating learning in business. These courses speed up the process of professionals learning basic data science skills.
The cloud and neural networks
The development of increasingly powerful computers that process and store greater amounts of data, in conjunction with ML algorithms, has brought about the need for a shift in the processing of training neural networks. Historically, training neural networks was carried out with central processing units (CPUs), however in 2017 Google released Tensor Processing Units (TPUs), faster and more powerful than CPUs.23 Google’s Cloud TPUs makes the ML that is behind Google Translate, Photos, Search, Assistant, and Gmail, available for businesses to supplement their data scientist’s efforts to achieve business breakthroughs.24
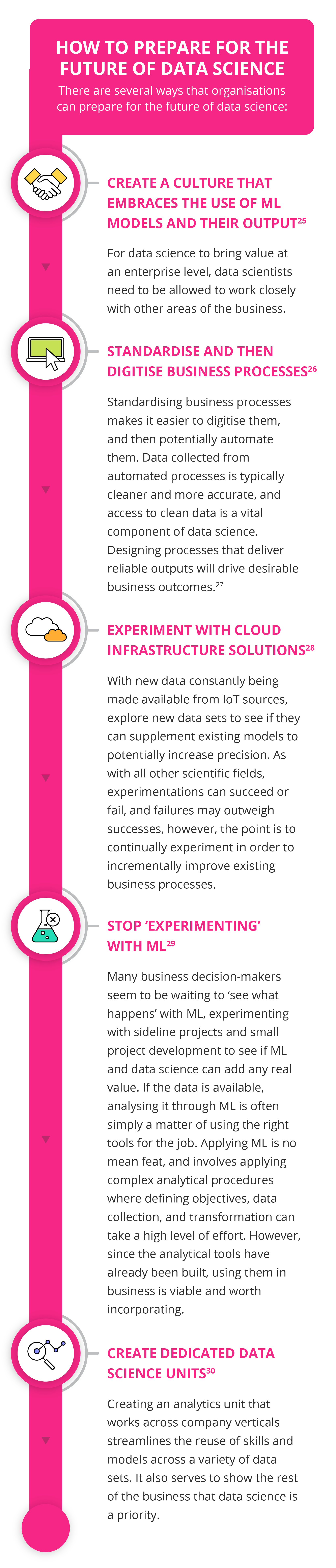
The need for the availability of clean, reliable data in order to produce meaningful business insights means that, for businesses who seek an advantage, data science is a skill set worth its weight in gold. Augmented by machine learning, AI, and cloud-based neural networks, data science will be in demand as long as there is a need for customer-centric insights and customer-driven data that could generate profit.
- 1 (2019). ‘Big data and AI executive survey 2019’. Retrieved from NewVantage.
- 2 Schatsky, D. et al. (Dec, 2018). ‘Democratizing data science to bridge the talent gap.’ Retrieved from Deloitte.
- 3 Appugliese, C. (Jun, 2018). ‘The future of data science’. Retrieved from SlideShare.
- 4 Ekne, H. (Nov, 2018). ‘The future of data science’. Retrieved from Towards Data Science.
- 5 Ekne, H. (Nov, 2018). ‘The future of data science’. Retrieved from Towards Data Science.
- 6 Ekne, H. (Nov, 2018). ‘The future of data science’. Retrieved from Towards Data Science.
- 7 (Nd). ‘Facebook newsroom’. Retrieved from Facebook. Accessed 29 April 2019.
- 8 (Nd). ‘YouTube for press’. Retrieved from YouTube. Accessed 29 April 2019.
- 9 Dhiraj, A. (Mar, 2019). ‘The 20 top most used social networking sites and apps in the world, 2019’. Retrieved from CEOWorld.
- 10 Ekne, H. (Nov, 2018). ‘The future of data science’. Retrieved from Towards Data Science.
- 11 Schatsky, D. et al. (Dec, 2018). ‘Democratizing data science to bridge the talent gap.’ Retrieved from Deloitte.
- 12 Ekne, H. (Nov, 2018). ‘The future of data science’. Retrieved from Towards Data Science.
- 13 Sasso, M. (May, 2018). ‘This is America’s hottest job’. Retrieved from Bloomberg.
- 14 Schatsky, D. et al. (Dec, 2018). ‘Democratizing data science to bridge the talent gap.’ Retrieved from Deloitte.
- 15 Gabernet, A. et al. (Aug, 2017). ‘Breaking the 80/20 rule: How data catalogs transform data scientists’ productivity’. Retrieved from IBM.
- 16 Rymer, J. (Aug, 2018). ‘Why you need to know about low-code, even if you’re not responsible for software delivery’. Retrieved from Forrester.
- 17 (Feb, 2018). ‘Pre-trained machine learning models for sentiment analysis and image detection’. Retrieved from Microsoft.
- 18 (Sep, 2018). ‘IBM largest ever AI toolset release is tailor made for 9 industries and professions’. Retrieved from IBM.
- 19 Dignan, L. (Mar, 2018). ‘Salesforce aims to bolster analytics for business users via natural language queries, easier visualization tools’. Retrieved from ZDNet.
- 20 Wiggers, K. (Sep, 2018). ‘Adobe Analytics can now automatically surface insights for you’. Retrieved from VentureBeat.
- 21 Baker, P. (Jul, 2018). ‘Microsoft Power BI’. Retrieved from PC Mag.
- 22 Marr, B. (Apr, 2018). ‘The 6 best free online artificial intelligence courses available today’. Retrieved from Forbes.
- 23 Ekne, H. (Nov, 2018). ‘The future of data science’. Retrieved from Towards Data Science.
- 24 (Nd). ‘Cloud TPU’. Retrieved from Google Cloud. Accessed 29 April 2019.
- 25 (Dec, 2018). ‘How to extract business value from data science: it’s all about the teamwork’. Retrieved from Forbes.
- 26 Ekne, H. (Nov, 2018). ‘The future of data science’. Retrieved from Towards Data Science.
- 27 (Dec, 2018). ‘How to extract business value from data science: it’s all about the teamwork’. Retrieved from Forbes.
- 28 Ekne, H. (Nov, 2018). ‘The future of data science’. Retrieved from Towards Data Science.
- 29 Dans, E. (Jul, 2019). ‘Stop experimenting with machine learning and start actually using it’. Retrieved from Forbes.
- 30 Ekne, H. (Nov, 2018). ‘The future of data science’. Retrieved from Towards Data Science.