What Is Algorithmic Trading?
Algorithmic trading refers to trade execution strategies typically used by Fund Managers to buy and sell large amounts of assets. These strategies rely on automated formulae to find market efficiencies and identify profitable patterns at a much higher frequency and speed than humans can achieve.
Machine learning for algorithmic trading
Automation is expected to be one of the eight megatrends that will significantly impact the future of work in the fourth industrial revolution. Machine learning (ML) and artificial intelligence (AI) have accelerated growth in almost every industry, from advertising and marketing to construction and finance. In algorithmic trading, machine learning is used to build predictive models that automatically identify patterns in large volumes of data. These models extract signals that can be used to inform your trade strategy.
How does algorithmic trading work?
In order to fully understand the nuances of algorithmic trading, it’s important to first understand the economic theories upon which algorithmic trading is based, as well as the nature of financial markets.
Traditional financial theory
In 1965, Eugene Francis Fama published a paper titled The Behavior of Stock Market Prices. His theory suggests that stock market price movements are random and therefore unpredictable.1 Five years later, he created the efficient market hypothesis, which states that stock always trades at fair value as all information about a company is already priced into its stock value.2 According to this theory, it would be impossible to “beat the market”.
Market participants
An efficient market relies on informed and rational market participants. For example, if Apple’s stock trades at £780 (its fair value) and an event occurs that changes its fair value to £600, traders will begin selling. The supply of Apple stock will increase, causing its price to drop. This will continue until the stock trades at fair value again. Some traders would’ve made a profit above market value, but this can’t occur consistently enough to model a winning algorithmic trading strategy.
Fundamental analysis uses both financial and economic information to calculate the fair value of a company’s stock. Historically, this was the primary method used by market participants to determine the impact of an event on stock prices. Trade would continue until stocks are driven back to fair market value.
Behavioural finance theory
We’ve learnt that efficient markets rely on rational market participants, but has every person you’ve met been completely rational? Behavioural finance recognises that individuals act irrationally and base decisions on information they’ve misinterpreted or misjudged. But how can behaviour be used as a base for building a programmed set of rules?
The crux is that many people have similar irrational reactions to certain events, making behaviour predictable. These patterns create statistical indicators that trigger the algorithm to take programmed action. The theory attempts to make sense of anomalies observed in market behaviour that cannot be accounted for by traditional models.
Forms of irrational behaviour in financial markets
Framing
The human brain needs context to make an informed decision. Depending on how information is presented, our interpretation of events may not be completely rational. For example, if you read the letters in the image below, the middle character appears to be a letter ‘B’. But if you read the numbers, the same character appears as a number ‘13’. This exercise demonstrates how a particular context can prompt a specific response.
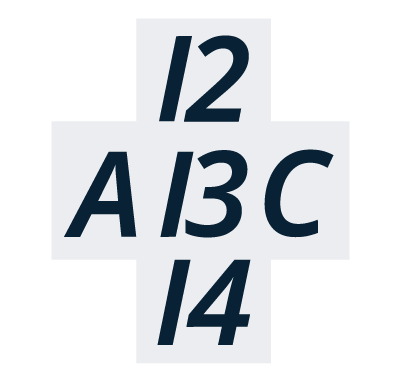
Psychologists Daniel Kahneman and Amos Tversky developed multiple theories concerning the cause and effect of irrational behaviour. Adverts proclaiming a discount or massive sale attempt to frame information in a certain way to entice a favourable response out of the public.3 Kahneman is the only non-economist to win a Nobel Prize in economics.
Bias
Over- and underreaction is a bias observed in the market. Using the Apple example, if the market was to overreact to the event that drove its stock price down, investors may think the stock’s fair value is below £600 (its actual fair value). If this could’ve been predicted, you could take advantage of this behaviour by simply buying the stock at its devalued price and waiting for the market to correct before selling.
Systematic trading to algorithmic trading
An algorithm reduces the risk of bias and framing in financial decision-making. But what rules must an algorithm follow in order to generate profit?
Systematic trading has been in place since the 1920s. In its earliest form, traders performed manual calculations based on charts and data which may have been posted weekly or collected in-person from the exchange.4 Algorithmic trading is a form of systematic trading and is the process of applying a predefined trading strategy (a set of rules) to the buying and selling of financial securities. Today, with the aid of machine learning, these calculations can be done in seconds.
Is algorithmic trading profitable?
Many forms of algorithmic trading strategies are geared towards taking advantage of small pricing discrepancies. They therefore require huge amounts of trades to generate profit. This high-frequency trading mechanism involves the frequent turnover of many small positions of security.
Market making
This is an example of high-frequency trading whereby firms attempt to take advantage of the bid-ask spread, i.e., the difference between what people are willing to sell the stock for, and what people are willing to buy it for. For example, if a firm purchases stock for £80 (the asking price) and sells it for £80.05 (the bid price), the price difference is so small that millions of trades will need to be made daily in order for this strategy to be effective.
One of the big challenges to market making is intense competition. More informed counter-parties such as hedge funds or similar trading companies could target the same market. Excellent infrastructure and the ability to act fast are key to remaining competitive in this space.
Market microstructure
It’s important to understand market microstructure if you want to build a successful high-frequency algorithmic trading strategy. Market microstructure looks at how markets are designed, how prices are formulated, how information is disclosed, as well as investor behaviour and transaction and timing costs. If you know what an order book is, you know prices are a product of supply and demand.
Algorithmic trading is currently estimated to account for 60% to 70% of equity trading in the U.S. and is expected to achieve a compound annual growth rate (CAGR) of 11.23% between 2021 and 2026.5
Testing an algorithmic trading model
It’s essential to test your model thoroughly to avoid losing capital under real-world conditions. Two primary methods are used to assess the accuracy of a predictive model.
- Out-of-sample testing involves withholding a portion of the sample data when the model is built and testing how accurately it can predict results for the withheld data.
- Backtesting uses historical market data to test models quickly and efficiently and provides immediate feedback on how these might have performed in the past.
Out-of-sample testing is crucial to determine how well the model performs on unseen data. If it can’t pass the out-of-sample tests, the model may need to be scrapped. It’s important not to over-fit the model – if you continue testing until it returns favourable results, you’ll have a model that looks good in tests but fails to succeed at generating profit.
There’s no clearly defined cause and effect for all market movements. When a trading strategy works, it’s not because it perfectly describes a mathematical principle. It’s because it captures a particular market characteristic, producing a positive return over time.
Algorithmic trading is not a get-rich-quick solution, but if you take the time to understand market behaviour and practice due diligence in terms of strategy validation, it’s possible to produce a winning set of rules that can be turned into a winning algorithmic trading strategy.
Invest in your trade and learn how you can harness the power of algorithmic trading
- 1 Fama, E. (Jan, 1965). ‘The Behavior of Stock-Market Prices’. Retrieved from JSTOR.
- 2 Fama, E. (May, 1970). ‘Efficient Capital Markets: A Review of Theory and Empirical Work’. Retrieved from JSTOR.
- 3 Tversky, A. & Kahneman, D. (Jan, 1981). ‘The Framing of Decisions and the Psychology of Choice’. Retrieved from Columbia Statistics.
- 4 Ruggiero Jnr., M. (May, 2014). ‘The long, winding tale of high-frequency systematic trading’. Retrieved from Futures.
- 5 (Nd). ‘Algorithmic Trading Market – Growth, Trends, Covid-19 Impact, and Forecasts (2022 – 2027)’. Retrieved from Mordor Intelligence. Accessed March 10, 2022.